We’re inviting SIFK affiliates and friends to share four things – books, films, essays, images, Twitter threads – that have pushed the thinking, teaching, and research in a new regular feature called Four Things on Formations. Our first round-up of four things comes from Alexander Campolo, a postdoctoral fellow at SIFK who studies data, sense, and knowledge.
Risk Society by Ulrich Beck (1986)
The experience of COVID has been disorienting in so many ways. An invisible virus rapidly spread across the globe, leaving havoc in its wake. In a prescient book from the 1980s Ulrich Beck gave us tools for thinking about these types of events, which he called “risks.” They emerged out of a paradoxical situation: modern science had given us unprecedented control over the natural world but was now starting to produce unintended consequences. The pandemic is a case in point. It was caused by human activity, whether through the destruction of animal habitats or risky virological research. And yet only human intervention—in the form of incredible advances in vaccine production and distribution—will end it. And, as Beck made clear, the highly unequal global distribution of vaccines shows that these scientific solutions are inseparable from politics. (Order Risk Society from Seminary Co-op)
The Normal and the Pathological by Georges Canguilhem (1943)
In The Normal and the Pathological, the philosopher Georges Canguilhem asks us to think about medical ideas that we often take for granted. What makes sickness different from health? A normal function different from a pathological one? His answer cuts against the grain of much of modern medicine. Canguilhem criticizes the use of numbers to characterize the normal and the pathological—how strange is it that we jump from “normal” to “overweight” when our body mass index moves from 24.9 kg/m2 to 25 kg/m2? Instead, vital norms like health express what works for an individual in a particular natural and social environment. The qualitative difference between sickness and health is not a biologically predetermined fact but a dynamic, even subjective matter of life’s values. From this perspective a “return to normal” following the pandemic would not involve a return to the past—Canguilhem believes that there is no reversibility when it comes to life—but the creation of new norms. (Order The Normal and the Pathological from Seminary Co-op)
Cloud Ethics by Louise Amoore (2020)
There is much interest in the ethical challenges posed by the rapid development of new AI and machine learning systems, which might determine your credit score, determine what you see on social media, or even make a medical diagnosis. An important first step in thinking ethically about algorithmic systems has been to mitigate their harmful effects and ensure that they are not discriminating against marginalized groups. Louise Amoore’s Cloud Ethics goes further, opening the door for more expansive ethical reflection on AI. Instead of asking “How ought the algorithm be arranged for a good society?” she asks, “How are algorithmic arrangements generating ideas of goodness, transgression, and what society ought to be?” In other words, how are algorithms changing how we conceive of society, ethics, and politics in the first place? One theme that runs through the work is that the focus on optimization in machine learning, which leads to a single output or prediction, limits the scope of our ethical possibilities. (Order Cloud Ethics from Seminary Co-op)
“Multimodal Neurons in Artificial Neural Networks,” OpenAI (2021), research presented on the AI publishing platform Distill
A group of researchers affiliated with OpenAI have developed an interesting approach to the problem of explainability or interpretability in machine learning. Put simply, although these systems make very accurate predictions or classifications, due to their complex structure, it is still difficult to understand how these predictions are made. The group at OpenAI addresses this problem by observing how neural networks behave. But this is no ordinary form of scientific observation. “Seeing” neural networks means producing data visualizations that “follow” an input forward and backward through a complicated sequence of mathematical operations. Although I am not sure that these visualizations will necessarily lead to meaningful scientific explanations, they are certainly thought-provoking.
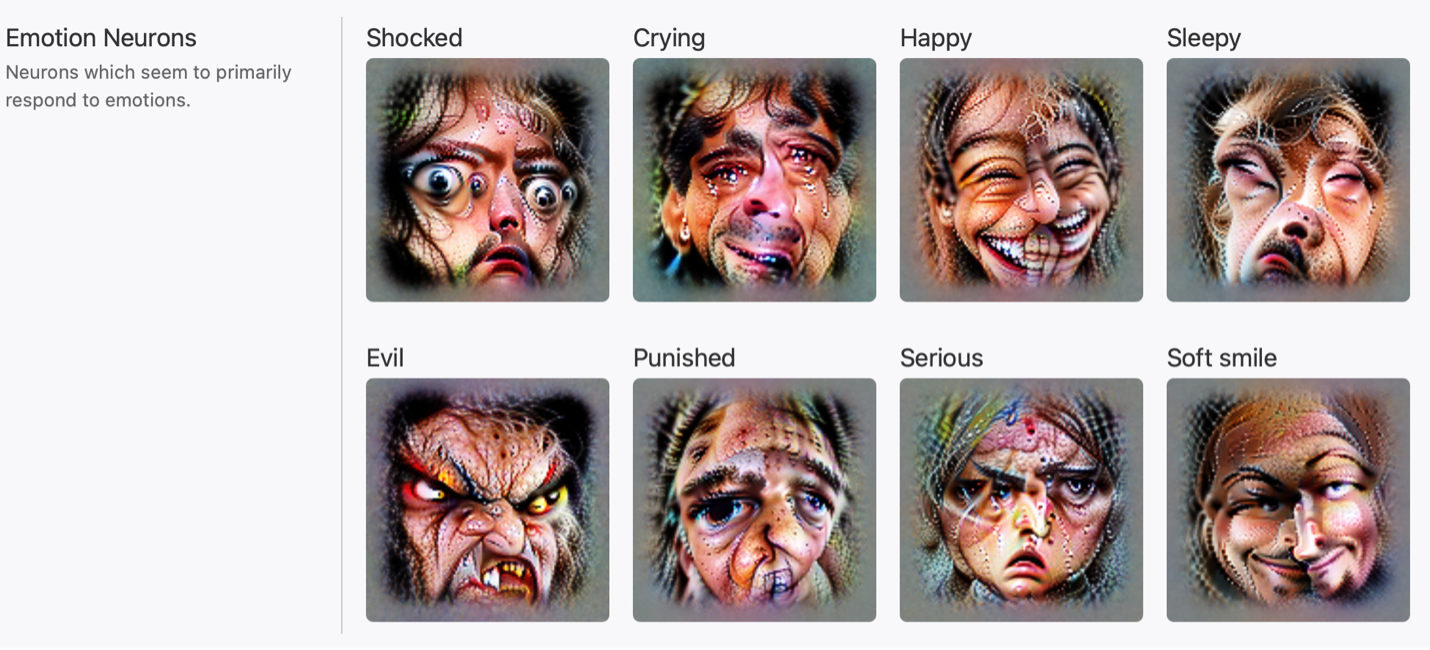
"The face facet shows facial expressions corresponding to different emotions, such as smiling, crying, or wide-eyed shock." A visualization from OpenAI.
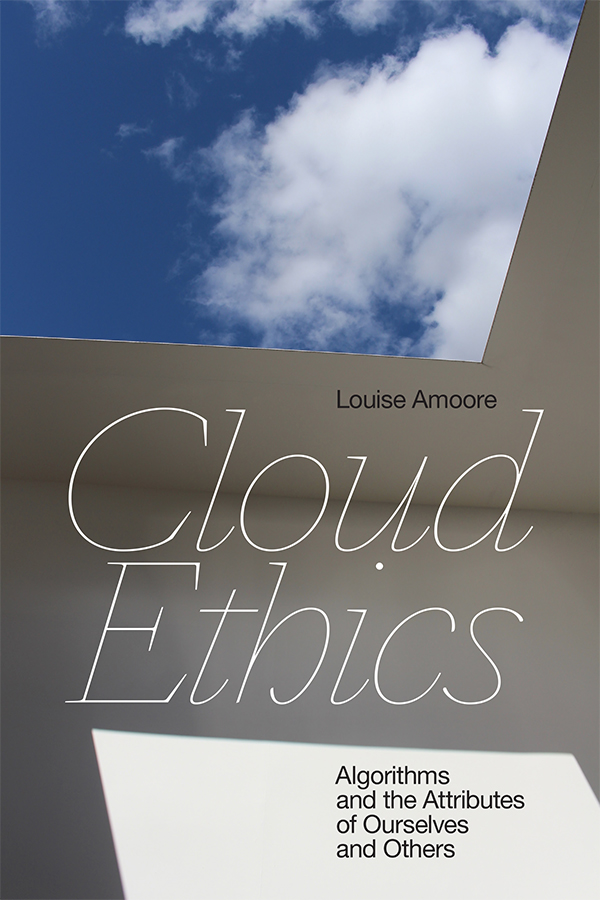
Cloud Ethics from Duke University Press.
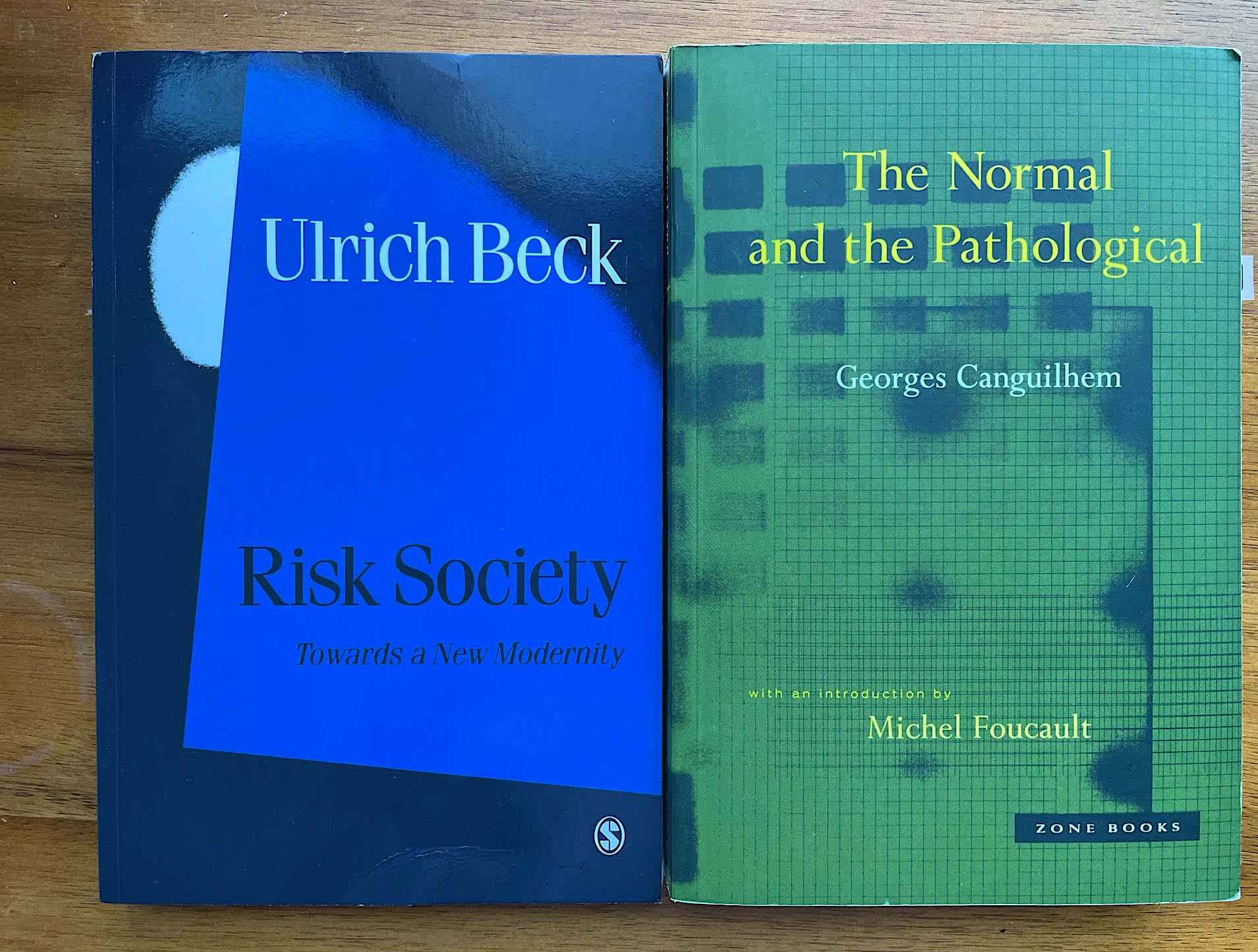